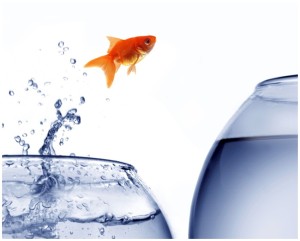
To say I’ve learnt a lot during my PhD candidature would be an understatement. From a single blank page, I now know more than most people in the world about my particular topic area. I understand the research process: from planning and designing a study; to conducting it; and then writing it up clearly – so that readers may be certain about what I did, how I did it, what I found, and why it’s important. I’ve met a variety of people from around the world, with similar interests and passions to me, and forged close friendships with many of them. And I’ve learnt that academia might well be the best career path in the world. After all, you get to choose your own research area; you have flexible working hours; you get to play around with ideas, concepts and data, and make new and often exciting discoveries; and you get to attend conferences (meaning you get to travel extensively, and usually at your employer’s expense), where you can socialise (often at open bars) under the guise of “networking”. Why, then, you might be wondering, would I want to leave all of that behind?
My journey through the PhD program has been fairly typical; I’ve gone through all of the usual stages. I’ve been stressed in the lead-up to (and during) my proposal defence. I’ve had imposter syndrome. And I’ve been worried about being scooped, and/or finding “that paper”, which presents the exact research I’m doing, but does it better than me. But now, as I begin my final year of the four year Australian program, I’m feeling comfortable with, and confident in, the work I’ve produced so far in my dissertation. And yet, I’m also disillusioned – because, for all of its positives, I’ve come to see academia as a broken institution.
That there are problems facing academic research is not news, especially in psychology. Stapel and Smeesters, researcher degrees of freedom and bias, (the lack of) statistical power and precision, the “replication crisis” and “theoretical amnesia”, social and behavioural priming: the list goes on. However, these problems are not altogether removed from one another; in fact, they highlight what I believe is a larger, underlying issue.
Academic research is no longer about a search for the truth
Stapel and Smeesters are two high profile examples of fraud, which represents an extreme exploitation of researcher degrees of freedom. But what makes any researcher “massage” their data? The bias towards publishing only positive results is no doubt a driving force. Does that excuse cases of fraud? Absolutely not. My point, however, is that there are clear pressures on the academic community to “publish or perish”. Consequently, academic research is largely an exercise in career development and promotion, and no longer (if, indeed, it ever was) an objective search for the truth.
For instance, the lack of statistical power evident in our field has been known for more than fifty years, with Cohen (1962) first highlighting the problem, and Rossi (1990) and Maxwell (2004) providing further prompts. Additionally, Cohen (1990; 1994) reminded us of the many issues associated with null-hypothesis significance testing – issues that were raised as far back as 1938 – and yet, it still remains the predominant form of data analysis for experimental researchers in the psychology field. To address these issues, Cohen (1994: 1002) suggested a move to estimation:
“Everyone knows” that confidence intervals contain all the information to be found in significance tests and much more. […] Yet they are rarely to be found in the literature. I suspect that the main reason they are not reported is that they are so embarrassingly large! But their sheer size should move us toward improving our measurement by seeking to reduce the unreliable and invalid part of the variance in our measures (as Student himself recommended almost a century ago). Also, their width provides us with the analogue of power analysis in significance testing – larger sample sizes reduce the size of confidence intervals as they increase the statistical power of NHST.
Twenty years later, and we’re finally starting to see some changes. Unfortunately, the field now has to suffer the consequences of being slow to change. Even if all our studies were powered at the conventional level of 80% (Cohen, 1988; 1992), they would still be imprecise; that is, the width of their 95% confidence intervals would be approximately ±70% of the point estimate or effect size (Goodman and Berlin, 1994). In practical terms, that means that if we used Cohen’s d as an effect size metric (for the standardised difference between two means), and we found that it was “medium” (that is, d = 0.50), the 95% confidence interval would range from 0.15 to 0.85. This is exactly what Cohen (1994) was talking about when he said the confidence intervals in our field are “so embarrassingly large”: in this case, the interval tells us that we can be 95% confident the true effect size is potentially smaller than “small” (0.20), larger than “large” (0.80), or somewhere in between. Remember, however, that many of the studies in our field are underpowered, which makes the findings even more imprecise than what is illustrated here; that is, the 95% confidence intervals are even wider. And so, I wonder: How many papers have been published in our field in the last twenty years, while we’ve been slow to change? And how many of these papers have reported results at least as meaningless as this example?
I suspect that part of the reason for the slow adoption of estimation techniques is due to the uncertainty they bring to the data. Significance testing is characterised by dichotomous thinking: an effect is either statistically significant or it is not. In other words, significance testing is seen as easier to conduct and analyse, relative to estimation; however, it does not allow for the same degree of clarity in our findings. By reporting confidence intervals (and highlighting uncertainty), we reduce the risk of committing one of the cardinal sins of consumer psychology: overgeneralisation. Furthermore, you may be surprised to learn that estimation is just as easy to conduct as significance testing, and even easier to report (because you can extrapolate greater meaning from your results).
Replication versus theoretical development
When you consider the lack of precision in our field, in conjunction with the magnitude of the problems of researcher degrees of freedom and publication bias, is it any wonder that so many replication attempts are unsuccessful? The issue of failed replications is then compounded further by the lack of theoretical development that takes place in our discipline, which creates additional problems. The incentive structure upon which the academic institution is situated implies that success (in the form of promotion and grants) comes to those who publish a high number of high quality papers (as determined by the journal in which they are published). As a result, we have a discipline that lacks both internal and external relevance, due to the multitude of standalone empirical findings that fail to address the full scope of consumer behaviour (Pham, 2013). In that sense, it seems to me that replication is at odds with theoretical development, when, in fact, the two should be working in tandem; that is, replication should guide theoretical development.
Over time, some of you may have observed (as I have) that single papers are now expected to “do more”. Papers will regularly report four or more experiments, in which they will identify an effect; perform a direct and/or conceptual replication; identify moderators and/or mediators and/or boundary conditions; and rule out alternative process accounts. I have heard criticism directed at this approach, usually from fellow PhD candidates, that there is an unfair expectation on the new generation of researchers to do more work to achieve what the previous generation did. In other words, that the seminal/classic papers in the field, upon which now-senior academics were awarded tenure, do less than what emerging and early career researchers are currently expected to do in their papers. I do not share this view that there is an issue of hypocrisy; rather, my criticism is that as the expectation that papers “do more” has grown, there is now less incentive for academics to engage in theoretical development. The “flashy” research is what gets noticed and, in turn, what gets its author(s) promoted and wins them grants. Why, then, would anyone waste their time trying to further develop an area of work that someone else has already covered so thoroughly – especially when, if you fail to replicate their basic effect, you will find it extremely difficult to publish in a flagship journal (where the “flashiest” research appears)?
This observation also begs the question: where has this expectation that papers “do more” come from? As other scientific fields (particularly the hard sciences) have reported more breakthroughs over time, I suspect that psychology has desired to keep up. The mind, however, in its intangibility, is too complex to allow for regular breakthroughs; there are simply too many variables that can come into effect, especially when behaviour is also brought into the equation. Such an issue is highlighted no more clearly than in the case of behavioural priming. Yet, with the development of a general theory of priming, researchers can target their efforts at identifying the varied and complex “unknown moderators” of the phenomenon and, in turn, design experiments that are more likely to replicate (Cesario, 2014). Consequently, the expectation for single papers to thoroughly explain an entire process is removed – and our replications can then do what they’re supposed to: enhance precision and uncover truth.
The system is broken
The psychology field seems resistant to regressing to simpler papers that take the time to develop theory, and contribute to knowledge in a cumulative fashion. Reviewers continue to request additional experiments, rather than to demand greater clarity from reported studies (for example, in the form of effect sizes and confidence intervals), and/or to encourage further theoretical development. Put simply, there is an implicit assumption that papers need to be “determining” when, in fact, they should be “contributing”. As Cumming (2014: 23) argues, it is important that a study “be considered alongside any comparable past studies and with the assumption that future studies will build on its contribution.”
In that regard, it would seem that the editorial/publication process is arguably the larger, underlying issue contributing (predominantly, though not necessarily solely) to the many problems afflicting academic research in psychology. But what is driving this issue? Could it be that the peer review process, which seems fantastic in theory, doesn’t work in practice? I believe that is certainly a possibility.
Something else I’ve come to learn throughout my PhD journey is that successful academic research requires mastery of several skills: you need to be able to plan your time; communicate your ideas clearly; think critically; explore issues from a “big picture” or macro perspective, as well as at the micro level; undertake conceptual development; design and execute studies; and be proficient at statistical analysis (assuming, of course, that you’re not an interpretive researcher). Interestingly, William Shockley, way back in 1957, posited that producing a piece of research involves clearing eight specific hurdles – and that these hurdles are essentially all equal. In other words, successful research calls for a researcher to be adept at each stage of the research process. However, in reality, it is often that the case that we are very adept (sometimes exceptional) at a few aspects, and merely satisfactory at others. The aim of the peer review process is to correct or otherwise improve the areas we are less adept at, which should – theoretically – result in a strong (sometimes exceptional) piece of research. Multiple reviewers evaluate a manuscript in an attempt to overcome these individual shortfalls; yet, look at the state of the discipline! The peer review process is clearly not working.
I’m not advocating abandoning the peer review process; I believe it is one of the cornerstones of scientific progress. What I am proposing, however, is for an adjustment to the system – and I’m not the first to do so. What if we, as has been suggested, move to a system of pre-registration? What if credit for publications in such a system were two-fold, with some going towards the conceptual development (resulting in the registered study), and some going towards the analysis and write-up? Such a system naturally lends itself to specialisation, so, what if we expected less of our researchers? That is, what if we were free to focus on those aspects of research that we’re good at (whether that’s, for example, conceptual development or data analysis), leaving our shortfalls to other researchers? What if the peer review process became specialised, with experts in the literature reviewing the proposed studies, and experts in data analysis reviewing the completed studies? This system also lends itself to collaboration and, therefore, to further skill development, because the experts in a particular aspect of research are well-recognised. The PhD process would remain more or less the same under this system, as it would allow emerging researchers to identify – honestly – their research strengths and weaknesses, before specialising after they complete grad school. There are, no doubt, issues with this proposal that I have not thought of, but to me, it suggests a stronger and more effective peer review process than the current one.
Unfortunately, I don’t believe these issues that I’ve outlined are going to change – at least not in a hurry, if the slow adoption of estimation techniques is anything to go by. For that reason, when I finish my PhD later this year, I will be leaving academia to pursue a career in market research, where obtaining truth from the data to deliver actionable insights to clients is of the utmost importance. Some may view this decision as synonymous with giving up, but it’s not a choice I’ve made lightly; I simply feel as though I have the opportunity to pursue a more meaningful career in research outside of academia – and I’m very much looking forward to the opportunities and challenges that lay ahead for me in industry.
For those who choose to remain in academia, it is your responsibility to promote positive change; that responsibility does not rest solely on the journals. It has been suggested that researchers boycott the flagship journals if they don’t agree with their policies – but that is really only an option for tenured professors, unless you’re willing to risk career self-sabotage (which, I’m betting, most emerging and early career researchers are not). The push for change, therefore, needs to come predominantly (though not solely) from senior academics, in two ways: 1) in research training, as advisors and supervisors of PhDs and post-docs; and 2) as reviewers for journals, and members of editorial boards. Furthermore, universities should offer greater support to their academics, to enable them to take the time to produce higher quality research that strives to discover the truth. Grant committees, also, may need to re-evaluate their criteria for awarding research grants, and focus more on quality and meaningful research, as opposed to research that is “flashy” and/or “more newsworthy”. And the next generation of academics (that is, the emerging and early career researchers) should familiarise themselves with these issues, so that they may make up their own minds about where they stand, how they feel, and how best to move forward; the future of the academic institution is, after all, in their hands.
Pingback: The Myth of Impartiality: Part 1 | OPRA's Learning Blog
Pingback: Viewpoint: Nudging Nudgers’ Nudges | :InDecision:
Pingback: The Myth of Impartiality: Part 1 | Paul Englert
Pingback: The Myth of Impartiality: Part 1 | Blog | OPRA Psychology Group